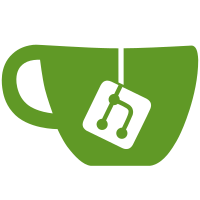
12 changed files with 661 additions and 59 deletions
@ -0,0 +1,61 @@
@@ -0,0 +1,61 @@
|
||||
""" Column name definitions """ |
||||
|
||||
# original, unmodified column names |
||||
COL_NAME_POS_X = "Pos.X" |
||||
COL_NAME_POS_Y = "Pos.Y" |
||||
COL_NAME_BKG_MEAN = "Bkg.Mean" |
||||
COL_NAME_SPOT_MEAN = "Spot.Mean" |
||||
COL_NAME_BKG_MEDIAN = "Bkg.Median" |
||||
COL_NAME_SPOT_MEDIAN = "Spot.Median" |
||||
COL_NAME_BKG_STDDEV = "Bkg.StdDev" |
||||
COL_NAME_SPOT_STDDEV = "Spot.StdDev" |
||||
COL_NAME_BKG_SUM = "Bkg.Sum" |
||||
COL_NAME_SPOT_SUM = "Spot.Sum" |
||||
COL_NAME_BKG_AREA = "Bkg.Area" |
||||
COL_NAME_SPOT_AREA = "Spot.Area" |
||||
COL_NAME_SPOT_SAT = "Spot.Sat. (%)" |
||||
COL_NAME_POS_NOM_X = "Pos.Nom.X" |
||||
COL_NAME_POS_NOM_Y = "Pos.Nom.Y" |
||||
|
||||
# replacement column names |
||||
COL_NAME_POS_ID = "Pos.Id" |
||||
COL_NAME_SPOT_FOUND = "Spot.Found" |
||||
COL_NAME_SPOT_DIAMETER = "Spot.Diameter" |
||||
|
||||
# additional column |
||||
COL_NAME_SPOT_OVERFLOW = "Spot.Overflow" |
||||
|
||||
# well information |
||||
COL_NAME_WELL_ROW = "Well.Row" |
||||
COL_NAME_WELL_COLUMN = "Well.Column" |
||||
|
||||
# parsed measurement parameter information |
||||
COL_NAME_PARAMETERS_CHANNEL = "Parameters.Channel" |
||||
COL_NAME_PARAMETERS_TIME = "Parameters.Time" |
||||
|
||||
# applied exposure info |
||||
COL_NAME_EXPOSURE_ID = "Exposure.Id" |
||||
COL_NAME_EXPOSURE_CHANNEL = "Exposure.Channel" |
||||
COL_NAME_EXPOSURE_TIME = "Exposure.Time" |
||||
|
||||
# normalized columns |
||||
COL_NAME_NORMALIZED_EXPOSURE_TIME = f"Normalized.{COL_NAME_EXPOSURE_TIME}" |
||||
COL_NAME_NORMALIZED_BKG_MEAN = f"Normalized.{COL_NAME_BKG_MEAN}" |
||||
COL_NAME_NORMALIZED_SPOT_MEAN = f"Normalized.{COL_NAME_SPOT_MEAN}" |
||||
COL_NAME_NORMALIZED_BKG_MEDIAN = f"Normalized.{COL_NAME_BKG_MEDIAN}" |
||||
COL_NAME_NORMALIZED_SPOT_MEDIAN = f"Normalized.{COL_NAME_SPOT_MEDIAN}" |
||||
COL_NAME_NORMALIZED_BKG_STDDEV = f"Normalized.{COL_NAME_BKG_STDDEV}" |
||||
COL_NAME_NORMALIZED_SPOT_STDDEV = f"Normalized.{COL_NAME_SPOT_STDDEV}" |
||||
COL_NAME_NORMALIZED_BKG_SUM = f"Normalized.{COL_NAME_BKG_SUM}" |
||||
COL_NAME_NORMALIZED_SPOT_SUM = f"Normalized.{COL_NAME_SPOT_SUM}" |
||||
|
||||
COLUMN_NORMALIZATION = { |
||||
COL_NAME_BKG_MEAN: COL_NAME_NORMALIZED_BKG_MEAN, |
||||
COL_NAME_SPOT_MEAN: COL_NAME_NORMALIZED_SPOT_MEAN, |
||||
COL_NAME_BKG_MEDIAN: COL_NAME_NORMALIZED_BKG_MEDIAN, |
||||
COL_NAME_SPOT_MEDIAN: COL_NAME_NORMALIZED_SPOT_MEDIAN, |
||||
COL_NAME_BKG_STDDEV: COL_NAME_NORMALIZED_BKG_STDDEV, |
||||
COL_NAME_SPOT_STDDEV: COL_NAME_NORMALIZED_SPOT_STDDEV, |
||||
COL_NAME_BKG_SUM: COL_NAME_NORMALIZED_BKG_SUM, |
||||
COL_NAME_SPOT_SUM: COL_NAME_NORMALIZED_SPOT_SUM, |
||||
} |
@ -0,0 +1,182 @@
@@ -0,0 +1,182 @@
|
||||
import numpy |
||||
|
||||
from .columns import ( |
||||
COL_NAME_POS_ID, |
||||
COL_NAME_WELL_ROW, |
||||
COL_NAME_SPOT_MEAN, |
||||
COL_NAME_EXPOSURE_ID, |
||||
COL_NAME_WELL_COLUMN, |
||||
COLUMN_NORMALIZATION, |
||||
COL_NAME_EXPOSURE_TIME, |
||||
COL_NAME_SPOT_OVERFLOW, |
||||
COL_NAME_PARAMETERS_TIME, |
||||
COL_NAME_EXPOSURE_CHANNEL, |
||||
COL_NAME_PARAMETERS_CHANNEL, |
||||
COL_NAME_NORMALIZED_EXPOSURE_TIME, |
||||
) |
||||
|
||||
|
||||
def _split_data_frame(data_frame, column): |
||||
""" splits a data frame on unique column values """ |
||||
values = data_frame[column].unique() |
||||
masks = {value: (data_frame[column] == value) for value in values} |
||||
return {value: data_frame[mask] for value, mask in masks.items()} |
||||
|
||||
|
||||
def _infer_exposure_from_parameters(data_frame): |
||||
""" infer the exposures from measurement parameters |
||||
|
||||
will raise a ValueError if the parameters contain NaNs |
||||
""" |
||||
df = data_frame # shorthand for cleaner code |
||||
|
||||
if ( |
||||
df[COL_NAME_PARAMETERS_CHANNEL].hasnans |
||||
or df[COL_NAME_PARAMETERS_TIME].hasnans |
||||
): |
||||
raise ValueError("Exposure Map: measurement parameters incomplete") |
||||
|
||||
df[COL_NAME_EXPOSURE_CHANNEL] = df[COL_NAME_PARAMETERS_CHANNEL] |
||||
df[COL_NAME_EXPOSURE_TIME] = df[COL_NAME_PARAMETERS_TIME] |
||||
return df |
||||
|
||||
|
||||
def apply_exposure_map(data_frame, exposure_map=None): |
||||
""" applies the parameters of a exposure map to the data frame |
||||
|
||||
exposure map: |
||||
keys: must be the same as the exposure ids, |
||||
values: objects with at least time and channel attributes |
||||
|
||||
if the exposure map is None, the values from the optionally parsed |
||||
measurement parameters are used. |
||||
|
||||
will raise an ValueError, if the provided exposure map does not map to the |
||||
exposure ids. |
||||
""" |
||||
|
||||
if exposure_map is None: |
||||
return _infer_exposure_from_parameters(data_frame) |
||||
|
||||
existing = set(data_frame[COL_NAME_EXPOSURE_ID].unique()) |
||||
provided = set(exposure_map.keys()) |
||||
if existing != provided: |
||||
raise ValueError( |
||||
f"Exposure Map differs from data frame: {provided} != {existing}" |
||||
) |
||||
|
||||
data_frame[COL_NAME_EXPOSURE_CHANNEL] = numpy.nan |
||||
data_frame[COL_NAME_EXPOSURE_TIME] = numpy.nan |
||||
for exposure_id, exposure_info in exposure_map.items(): |
||||
mask = data_frame[COL_NAME_EXPOSURE_ID] == exposure_id |
||||
data_frame.loc[mask, COL_NAME_EXPOSURE_CHANNEL] = exposure_info.channel |
||||
data_frame.loc[mask, COL_NAME_EXPOSURE_TIME] = exposure_info.time |
||||
return data_frame |
||||
|
||||
|
||||
def _check_overflow_limit(data_frame, column=COL_NAME_SPOT_MEAN, limit=0.5): |
||||
""" add overflow info, based on column and limit """ |
||||
data_frame[COL_NAME_SPOT_OVERFLOW] = data_frame[column] > limit |
||||
return data_frame |
||||
|
||||
|
||||
def reduce_overflow(data_frame, column=COL_NAME_SPOT_MEAN, limit=0.5): |
||||
""" reduces the data set per channel, eliminating overflowing spots """ |
||||
data_frame = _check_overflow_limit(data_frame, column, limit) |
||||
|
||||
split_frames = _split_data_frame(data_frame, COL_NAME_EXPOSURE_CHANNEL) |
||||
|
||||
return { |
||||
channel_id: _reduce_overflow_in_channel(channel_frame) |
||||
for channel_id, channel_frame in split_frames.items() |
||||
} |
||||
|
||||
|
||||
def _reduce_overflow_in_channel(channel_frame): |
||||
""" does the heavy lifting for reduce_overflow """ |
||||
|
||||
split_frames = _split_data_frame(channel_frame, COL_NAME_EXPOSURE_TIME) |
||||
|
||||
if len(split_frames) == 1: |
||||
# shortcut, if there is only one exposure in the channel |
||||
return channel_frame |
||||
|
||||
exposure_times = sorted(split_frames.keys(), reverse=True) |
||||
max_time, *rest_times = exposure_times |
||||
|
||||
multi_index = [COL_NAME_WELL_ROW, COL_NAME_WELL_COLUMN, COL_NAME_POS_ID] |
||||
result_frame = split_frames[max_time].set_index(multi_index) |
||||
|
||||
for next_time in rest_times: |
||||
mask = result_frame[COL_NAME_SPOT_OVERFLOW] == True # noqa: E712 |
||||
next_frame = split_frames[next_time].set_index(multi_index) |
||||
result_frame.loc[mask] = next_frame.loc[mask] |
||||
|
||||
return result_frame.reset_index() |
||||
|
||||
|
||||
def _infer_normalization_map(split_data_frames): |
||||
""" extract a time normalization map from split data frames """ |
||||
return { |
||||
key: frame[COL_NAME_EXPOSURE_TIME].max() |
||||
for key, frame in split_data_frames.items() |
||||
} |
||||
|
||||
|
||||
def normalize_exposure_time(split_data_frames, normalization_map=None): |
||||
""" add time normalized values to the split data frames |
||||
|
||||
normalization_map: |
||||
keys: channel identifier (e.g. "Cy5") |
||||
values: target exposure time for normalization |
||||
|
||||
If normalization_map is None, the max exposure time per channel is used |
||||
""" |
||||
complete_map = _infer_normalization_map(split_data_frames) |
||||
if normalization_map is not None: |
||||
complete_map.update(normalization_map) |
||||
|
||||
return { |
||||
key: _normalize_exposure(frame, complete_map[key]) |
||||
for key, frame in split_data_frames.items() |
||||
} |
||||
|
||||
|
||||
def _normalize_exposure(channel_frame, normalized_time): |
||||
""" add time normalized values to a channel data frames """ |
||||
channel_frame[COL_NAME_NORMALIZED_EXPOSURE_TIME] = normalized_time |
||||
|
||||
for original_col, normalized_col in COLUMN_NORMALIZATION.items(): |
||||
channel_frame[normalized_col] = ( |
||||
channel_frame[original_col] / channel_frame[COL_NAME_EXPOSURE_TIME] |
||||
) * channel_frame[COL_NAME_NORMALIZED_EXPOSURE_TIME] |
||||
|
||||
return channel_frame |
||||
|
||||
|
||||
def normalize_measurement( |
||||
data_frame, |
||||
exposure_map=None, |
||||
normalization_map=None, |
||||
overflow_column=COL_NAME_SPOT_MEAN, |
||||
overflow_limit=0.5, |
||||
): |
||||
""" augment normalize the measurement exposures |
||||
|
||||
exposure map: |
||||
keys: must be the same as the exposure ids, |
||||
values: objects with at least time and channel attributes |
||||
if the exposure map is None, the values from the optionally parsed |
||||
measurement parameters are used. |
||||
|
||||
normalization_map: |
||||
keys: channel identifier (e.g. "Cy5") |
||||
values: target exposure time for normalization |
||||
If normalization_map is None, the max exposure time per channel is used |
||||
""" |
||||
|
||||
exposure_data_frame = apply_exposure_map(data_frame, exposure_map) |
||||
split_data_frames = reduce_overflow( |
||||
exposure_data_frame, overflow_column, overflow_limit |
||||
) |
||||
return normalize_exposure_time(split_data_frames, normalization_map) |
@ -0,0 +1,294 @@
@@ -0,0 +1,294 @@
|
||||
from collections import namedtuple |
||||
|
||||
import pandas |
||||
import pytest |
||||
|
||||
from .conftest import EXAMPLE_DIR_WO_PARAMS, EXAMPLE_DIR_WITH_PARAMS |
||||
|
||||
ExposureSetting = namedtuple("ExposureSetting", ["channel", "time"]) |
||||
|
||||
|
||||
def test_split_data_frame(example_dir): |
||||
from sensospot_data.parser import process_folder |
||||
from sensospot_data.normalisation import _split_data_frame |
||||
|
||||
data_frame = process_folder(example_dir / EXAMPLE_DIR_WITH_PARAMS) |
||||
|
||||
result = _split_data_frame(data_frame, "Well.Row") |
||||
|
||||
assert set(result.keys()) == set("ABC") |
||||
for key, value_df in result.items(): |
||||
assert set(value_df["Well.Row"].unique()) == {key} |
||||
|
||||
|
||||
def test_infer_exposure_from_parameters(example_dir): |
||||
from sensospot_data.parser import process_folder |
||||
from sensospot_data.normalisation import _infer_exposure_from_parameters |
||||
|
||||
data_frame = process_folder(example_dir / EXAMPLE_DIR_WITH_PARAMS) |
||||
result = _infer_exposure_from_parameters(data_frame) |
||||
|
||||
assert all(result["Exposure.Channel"] == result["Parameters.Channel"]) |
||||
assert all(result["Exposure.Time"] == result["Parameters.Time"]) |
||||
|
||||
|
||||
def test_infer_exposure_from_parameters_raises_error(example_dir): |
||||
from sensospot_data.parser import process_folder |
||||
from sensospot_data.normalisation import _infer_exposure_from_parameters |
||||
|
||||
data_frame = process_folder(example_dir / EXAMPLE_DIR_WO_PARAMS) |
||||
|
||||
with pytest.raises(ValueError) as excinfo: |
||||
_infer_exposure_from_parameters(data_frame) |
||||
|
||||
assert str(excinfo.value).startswith("Exposure Map: measurement") |
||||
|
||||
|
||||
def test_apply_exposure_map(example_dir): |
||||
from sensospot_data.parser import process_folder |
||||
from sensospot_data.normalisation import apply_exposure_map |
||||
|
||||
exposure_map = { |
||||
1: ExposureSetting("Cy3", 100), |
||||
2: ExposureSetting("Cy5", 15), |
||||
3: ExposureSetting("Cy5", 150), |
||||
} |
||||
|
||||
data_frame = process_folder(example_dir / EXAMPLE_DIR_WITH_PARAMS) |
||||
result = apply_exposure_map(data_frame, exposure_map) |
||||
|
||||
for key, value in exposure_map.items(): |
||||
mask = result["Exposure.Id"] == key |
||||
partial = result.loc[mask] |
||||
assert set(partial["Exposure.Channel"].unique()) == {value.channel} |
||||
assert set(partial["Exposure.Time"].unique()) == {value.time} |
||||
|
||||
|
||||
def test_apply_exposure_map_raises_error(example_dir): |
||||
from sensospot_data.parser import process_folder |
||||
from sensospot_data.normalisation import apply_exposure_map |
||||
|
||||
exposure_map = { |
||||
1: ExposureSetting("Cy3", 100), |
||||
2: ExposureSetting("Cy5", 15), |
||||
"X": ExposureSetting("Cy5", 150), |
||||
} |
||||
|
||||
data_frame = process_folder(example_dir / EXAMPLE_DIR_WITH_PARAMS) |
||||
|
||||
with pytest.raises(ValueError) as excinfo: |
||||
apply_exposure_map(data_frame, exposure_map) |
||||
|
||||
assert str(excinfo.value).startswith("Exposure Map differs") |
||||
|
||||
|
||||
def test_apply_exposure_map_from_parameters(example_dir): |
||||
from sensospot_data.parser import process_folder |
||||
from sensospot_data.normalisation import apply_exposure_map |
||||
|
||||
data_frame = process_folder(example_dir / EXAMPLE_DIR_WITH_PARAMS) |
||||
result = apply_exposure_map(data_frame, None) |
||||
|
||||
assert all(result["Exposure.Channel"] == result["Parameters.Channel"]) |
||||
assert all(result["Exposure.Time"] == result["Parameters.Time"]) |
||||
|
||||
|
||||
def test_apply_exposure_map_from_parameters_raises_error(example_dir): |
||||
from sensospot_data.parser import process_folder |
||||
from sensospot_data.normalisation import apply_exposure_map |
||||
|
||||
data_frame = process_folder(example_dir / EXAMPLE_DIR_WO_PARAMS) |
||||
|
||||
with pytest.raises(ValueError) as excinfo: |
||||
apply_exposure_map(data_frame, None) |
||||
|
||||
assert str(excinfo.value).startswith("Exposure Map: measurement") |
||||
|
||||
|
||||
def test_check_overflow_limit_defaults(): |
||||
from sensospot_data.normalisation import _check_overflow_limit |
||||
|
||||
data_frame = pandas.DataFrame(data={"Spot.Mean": [0.1, 0.5, 0.6]}) |
||||
|
||||
result = _check_overflow_limit(data_frame) |
||||
|
||||
assert list(result["Spot.Overflow"]) == [False, False, True] |
||||
|
||||
|
||||
def test_check_overflow_limit_custom_limit(): |
||||
from sensospot_data.normalisation import _check_overflow_limit |
||||
|
||||
data_frame = pandas.DataFrame(data={"Spot.Sat": [4, 2, 3, 4]}) |
||||
|
||||
result = _check_overflow_limit(data_frame, "Spot.Sat", 2) |
||||
|
||||
assert list(result["Spot.Overflow"]) == [True, False, True, True] |
||||
|
||||
|
||||
def test_reduce_overflow_in_channel(normalization_data_frame): |
||||
from sensospot_data.normalisation import ( |
||||
_reduce_overflow_in_channel, |
||||
_check_overflow_limit, |
||||
) |
||||
|
||||
data_frame = _check_overflow_limit( |
||||
normalization_data_frame, "Saturation", 1 |
||||
) |
||||
result = _reduce_overflow_in_channel(data_frame) |
||||
|
||||
sorted_results = result.sort_values( |
||||
by=["Well.Row", "Well.Column", "Pos.Id"] |
||||
) |
||||
|
||||
assert list(sorted_results["Value"]) == [ |
||||
1, |
||||
2, |
||||
3, |
||||
1, |
||||
10, |
||||
10, |
||||
10, |
||||
10, |
||||
100, |
||||
100, |
||||
100, |
||||
100, |
||||
] |
||||
|
||||
|
||||
def test_reduce_overflow_in_channel_shortcut(normalization_data_frame): |
||||
from sensospot_data.normalisation import ( |
||||
_reduce_overflow_in_channel, |
||||
_check_overflow_limit, |
||||
) |
||||
|
||||
normalization_data_frame["Exposure.Time"] = 1 |
||||
|
||||
data_frame = _check_overflow_limit( |
||||
normalization_data_frame, "Saturation", 1 |
||||
) |
||||
result = _reduce_overflow_in_channel(data_frame) |
||||
|
||||
assert result is data_frame |
||||
|
||||
|
||||
def test_reduce_overflow(normalization_data_frame): |
||||
from sensospot_data.normalisation import reduce_overflow |
||||
|
||||
result = reduce_overflow(normalization_data_frame, "Saturation", 1) |
||||
|
||||
assert "Cy5" in result |
||||
|
||||
sorted_results = result["Cy5"].sort_values( |
||||
by=["Well.Row", "Well.Column", "Pos.Id"] |
||||
) |
||||
|
||||
assert list(sorted_results["Value"]) == [ |
||||
1, |
||||
2, |
||||
3, |
||||
1, |
||||
10, |
||||
10, |
||||
10, |
||||
10, |
||||
100, |
||||
100, |
||||
100, |
||||
100, |
||||
] |
||||
|
||||
|
||||
def test_infer_normalization_map(normalization_data_frame): |
||||
from sensospot_data.normalisation import ( |
||||
_infer_normalization_map, |
||||
_split_data_frame, |
||||
) |
||||
|
||||
normalization_data_frame.loc[5, "Exposure.Channel"] = "Cy3" |
||||
split_frames = _split_data_frame( |
||||
normalization_data_frame, "Exposure.Channel" |
||||
) |
||||
|
||||
result = _infer_normalization_map(split_frames) |
||||
|
||||
assert result == {"Cy3": 25, "Cy5": 50} |
||||
|
||||
|
||||
def test_normalize_exposure(normalization_data_frame): |
||||
from sensospot_data.normalisation import ( |
||||
_normalize_exposure, |
||||
reduce_overflow, |
||||
) |
||||
from sensospot_data.columns import COLUMN_NORMALIZATION |
||||
|
||||
reduced = reduce_overflow(normalization_data_frame, "Saturation", 1) |
||||
result = _normalize_exposure(reduced["Cy5"], 100) |
||||
|
||||
sorted_results = result.sort_values( |
||||
by=["Well.Row", "Well.Column", "Pos.Id"] |
||||
) |
||||
expected_values = [2, 8, 30, 2, 20, 20, 20, 20, 200, 200, 200, 200] |
||||
|
||||
for normalized_col in COLUMN_NORMALIZATION.values(): |
||||
list(sorted_results[normalized_col]) == expected_values |
||||
|
||||
|
||||
def test_normalize_exposure_time(normalization_data_frame): |
||||
from sensospot_data.normalisation import ( |
||||
normalize_exposure_time, |
||||
reduce_overflow, |
||||
) |
||||
|
||||
reduced = reduce_overflow(normalization_data_frame, "Saturation", 1) |
||||
result = normalize_exposure_time(reduced, {"Cy5": 100, "Cy3": 0}) |
||||
|
||||
assert "Cy5" in result |
||||
|
||||
sorted_results = result["Cy5"].sort_values( |
||||
by=["Well.Row", "Well.Column", "Pos.Id"] |
||||
) |
||||
expected_values = [2, 8, 30, 2, 20, 20, 20, 20, 200, 200, 200, 200] |
||||
|
||||
assert list(sorted_results["Normalized.Spot.Mean"]) == expected_values |
||||
|
||||
|
||||
def test_normalize_exposure_time_infered_map(normalization_data_frame): |
||||
from sensospot_data.normalisation import ( |
||||
normalize_exposure_time, |
||||
reduce_overflow, |
||||
) |
||||
|
||||
reduced = reduce_overflow(normalization_data_frame, "Saturation", 1) |
||||
result = normalize_exposure_time(reduced) |
||||
|
||||
assert "Cy5" in result |
||||
|
||||
sorted_results = result["Cy5"].sort_values( |
||||
by=["Well.Row", "Well.Column", "Pos.Id"] |
||||
) |
||||
expected_values = [1, 4, 15, 1, 10, 10, 10, 10, 100, 100, 100, 100] |
||||
|
||||
assert list(sorted_results["Normalized.Spot.Mean"]) == expected_values |
||||
|
||||
|
||||
def test_normalize_measurement(example_dir): |
||||
from sensospot_data.normalisation import normalize_measurement |
||||
from sensospot_data.parser import process_folder |
||||
|
||||
sub_dir = example_dir / EXAMPLE_DIR_WITH_PARAMS |
||||
data_frame = process_folder(sub_dir) |
||||
|
||||
exposure_map = { |
||||
1: ExposureSetting("Cy3", 100), |
||||
2: ExposureSetting("Cy5", 15), |
||||
3: ExposureSetting("Cy5", 150), |
||||
} |
||||
normalization_map = {"Cy5": 25} |
||||
|
||||
result = normalize_measurement(data_frame, exposure_map, normalization_map) |
||||
cy3_df, cy5_df = result["Cy3"], result["Cy5"] |
||||
|
||||
assert set(result.keys()) == {"Cy3", "Cy5"} |
||||
assert cy3_df["Normalized.Exposure.Time"].unique() == 100 |
||||
assert cy5_df["Normalized.Exposure.Time"].unique() == 25 |
Loading…
Reference in new issue